Projects available
Applications are invited for the AI for Drug Discovery Programme for the available projects listed below.
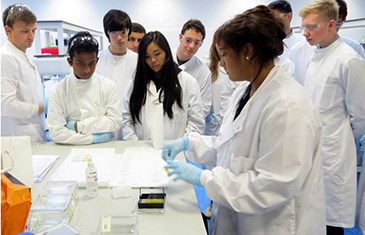
We are delighted in this cross-faculty and cross-disciplinary training programme with our industrial partners to train the next generation of drug discovery researchers— Professor Michael Barnes, Professor of Bioinformatics. The William Harvey Research Institute, Faculty of Medicine and Dentistry
Please see below details of the available projects for the 2025-26 intake. Please note the application deadline as listed per project and ensure your application is submitted in-time. Available projects are open to candidates who meet the UKRI terms and conditions, and are classed as Home for tuition fee purposes. Further details in the project descriptions below.
Each project has a supervisor based at Queen Mary, and engagement from Industry, including the option for a placement. The level of industry engagement varies depending on the nature of the project. We suggest you review each project description to learn more about the proposed research. Once you have identified your top project, you can submit an application via the Apply page. Note, you will be asked to identify your chosen project, and a maximum of 1 other project; you cannot apply for more than 2 projects, so we recommend you consider your choice carefully, ensuring that it is the right fit for you and your research aspirations.
Points to consider when reviewing projects:
- Is the project a good fit for my research experience to-date, and my research interests?
- Do I have the necessary background knowledge, or could I reasonably acquire this through targeted training on the programme?
- What attracts me to this project, and which part of the project most excites me?
- Does the supervisory team seem a good fit for me, and what makes me want to work with them?
Development of Generalisable Multi-Modal ML Models for Digital Pathology
Development of Generalisable Multi-Modal ML Models for Digital Pathology
Application Deadline - July 6th 2025
Bio-AI Health has a strong focus on development of ML based models that can help in patient screening and selection for clinical development. Our models tend to focus on detection of key biomarkers of interest which are typically detected via some type of molecular or chemical test on a digital H&E image. There are numerous publications which show the potential of such technology, however most of these models lack enough generalization for them to be successfully incorporated in real world practice. Models tend to perform well when trained on specific data sets but fail when transferred to new labs or new cohorts.
Our aim is to leverage molecular (or other) signals from these biomarkers and integrate them into our computer vision models in such a way that will allow for the model to focus on the signal of interest and lose any staining or processing bias. This will allow us to train a generalizable model that can be effective across multiple labs / cohorts of data. Additionally, our aim is to leverage the images plus molecular data for the development of the model, therefore vectorizing the molecular signal in such a way that we will be able to test and run the model on image data only. Use of technology such as 10x Visium will allow for precise characterization of signal with a spatial component.
The main objectives for the PhD project will be to:
- Model generalization across cohorts for digital pathology
- Multimodal integration of digital images and molecular signals generated from a RNAseq (or other omic data)
- Leverage spatial transcriptomics data and combine with computer vision for model development and optimization
- Help come up with innovative approaches for development of robust generalizable models on digital pathology data for personalized medicine
- Help with the integration of foundational pathology models for the fine tuning of specific tasks that allows for model generalization
Candidates will need a strong background in deep learning, particularly computer vision, and a knowledge of omics including transcriptomics. Familiarity with histopathology images, knowledge of model generalisation techniques and experience working with multimodal AI or graph neural networks will be advantageous.
Eligibility and Applying
This project is part of the UKRI/BBSRC AI for Drug Discovery Progamme, and successful candidates will join a cohort of students working on complementary projects in the AI for Drug Discovery space.
We are looking for highly motivated individuals who are passionate about contributing to new discoveries in drug discovery bioscience through the application of the latest techniques in AI and data science. Ideal candidates will have a grounding in both a natural science and data science, e.g. through a Master's degree or work experience in a subject such as bioinformatics or computational chemistry. Alternatively, you may have, for example, a first-class degree in computer science followed by biochemistry experience, or vice versa (qualifications and evidence thereof must be obtained before October 2025). You will be confident in performing data wrangling and analysis in a language such as Python, R or C++. Effective communication skills are essential.
We particularly encourage students from groups that are currently underrepresented in postgraduate science research, including black and minority ethnic students and those from a socio-economically disadvantaged background.
The Studentship will cover UK tuition fees, UKRI stipend (currently £21,637) and a consumable allowance for a period of 4-years (pro-rata for part-time), and is open to candidates who meet the UKRI eligibility criteria. This typically means the candidate will have unrestricted access on how long they can remain in the UK (i.e. are a British National, have settled, or pre-settled status, have indefinite leave to remain etc.) and have been living in the UK for the 3 years immediately prior to studentship starting. Candidates who would be classed as International are unfortunately not eligible for this opportunity.
Visit the Apply Pages for further details on how to submit an application.
Supervisors:
Dr Vivek Singh - Lecturer in Digital Pathology, Barts Cancer Institute, QMUL
Dr Alan Jerusalmi - Chief Innovation Officer & Co-Founder, Bio-AI Health
Project Partner: Bio-AI Health